Health insurance
Recent Patent Trial and Appeal Board Approaches to Patent Claims on Medical Technology Implementing AI
[ad_1]
First published by ALM / Law.com in The Intellectual Property Strategist.
I. INTRODUCTION
This article briefly analyzes recent Patent Trial and Appeal Board (“PTAB”) decisions on medical technology incorporating artificial intelligence (AI). Each decision involves reversal of a prior art rejection and contrasts with the other decisions on subject matter eligibility. The innovations at issue relate to a range of medical technologies concerning anatomical location-based machine learning models to predict pathological abnormalities, operation of a robotic surgical device prompted by an intra-operative surgical recommendation, and polygenic models to predict characteristics of individuals based on genetic variants. The decisions reveal different PTAB approaches and results that can inform prosecution and appeal strategies.
II. PTAB DECISIONS
A. Reversal of Prior Art Rejection, No Alice Issue
In Ex Parte Lyman, Appeal No. 2022-002753 (PTAB Sept. 25, 2023), an appeal to the PTAB involved technology relating to AI-based analysis of medical scans generated by medical imaging devices. The technology utilizes image data from a medical scan to train machine learning models based on datasets of medical scans and associated labels. The labels relate to pathological abnormality classes, such as atelectasis, effusion, mass, pneumonia, consolidation, emphysema, pleural thickening, cardiomegaly, infiltration, nodule, pneumothorax, edema, fibrosis, and hernia. In relevant part, the claims recited training a generical machine learning model based on a set of medical scan data and training a plurality of anatomical location-based machine learning models by performance of a fine tuning step on the generic machine learning model. In the claims, mutually exclusive subsets of the medical scan data are generated based on different anatomical locations. The claims further recited that, with respect to fine tuning, a subset of the medical scan data relating to an anatomical location is utilized to train a corresponding machine learning model associated with the anatomical location.
The sole issue on appeal was the correctness of the rejection of the claims under section 103. In particular, a prior art reference relied upon by the examiner disclosed a single process to train three different models, each model trained using a corresponding subset of data. However, the PTAB noted that the prior art reference failed to teach training of the claimed generic machine learning model and training of location-based models using distinct subsets of the data used to train the generic machine learning model. As a result, the PTAB reversed the prior art rejection of the claims.
In this case, no Alice issues were brought to or raised by the PTAB. Notably, an early version of the claims recited, inter alia, generating a generic model by performance of a training step and generating a plurality of location-based models by performance of a fine tuning step. During prosecution, the examiner rejected this version of the claims, finding that the claims covered a method of organizing human activity. In response, the applicant argued that the claims did not involve people at all, much less managing personal behavior or relationships between people, and that the claims recited a practical application in the form of an improvement to the technology of medical imaging. Unpersuaded by these arguments, the examiner noted that, under a broadest reasonable interpretation, the claims did not recite use of a machine learning model and accordingly urged claim amendments to recite “training and use” of a machine learning model. After a claim amendment heeding that advice, the Alice rejection was withdrawn before the appeal.
B. Reversal of Prior Art Rejection, Unexpected Alice Issue
Ex parte Couture, Appeal No. 2023-002437 (PTAB Sept. 28, 2023) was an appeal involving a machine learning model trained to provide an intra-operative surgical recommendation to a surgeon operating a robotic surgical device. The robotic surgical device can be used to perform, for example, a knee arthroplasty, a hip arthroplasty, or a shoulder arthroplasty. The claims recited a system including a robotic surgical device and a machine learning engine capable of generating a machine learning model trained based on training data. The training data as claimed includes a set of robotic surgical procedure weights based on robotic surgical operation data associated with a positive surgical procedure outcome. In the claims, the robotic surgical procedure weights are associated with surgical outcome metrics. The applicant appealed final rejection of the claims under section 103, the only outstanding rejection.
The entire PTAB panel found that the recitation of training data distinguished the claims over the prior art references. A key prior art reference disclosed a machine learning system and procedure outcome data associated with a result from a surgical procedure, including whether the result was positive or negative. However, the panel found no disclosure in the reference that the procedure outcome data was based on the claimed robotic surgical procedure weights. The panel thus concluded that the examiner erred and reversed the rejection of the claims.
One administrative patent judge concurred in the decision, sua sponte asserting that claim 1 failed the Alice test. The APJ acknowledged but faulted the examiner’s withdrawal of section 101 rejections in prosecution based on certain claim amendments. The claim amendments recited a surgical device and a trained machine learning model to determine a surgical prediction and, according to the examiner, constituted integration of an abstract idea into a practical application. As to step 1 of the Alice test, the APJ asserted that all device elements were generically claimed, including a generically claimed machine learning engine. Despite the recited detail regarding the machine learning model trained on specific training data including robotic surgical procedure weights based on robotic surgical operation data, the APJ characterized the claims as covering the use of any type of machine learning and not reciting technological implementation details. As to step 2, the APJ argued that the applicant could not contend that it invented a machine learning engine, citing mention in the specification to techniques such as artificial neural networks, Bayesian networks, instance-based learning, support vector machines, and decision trees. Again, without addressing the detailed recitation in the claims regarding the machine learning model trained on specific training data and associated generation of a robotic surgical procedure prediction, the APJ contended that use of a nondescript machine learning engine with a robotic device was associated with the abstract idea itself, not a technological improvement. The APJ did not discuss whether more express recital of training and use of a specific machine learning model trained with the claimed training data including the robotic surgical procedure weights would constitute an improvement or inventive concept to satisfy the second prong of step 2A or step 2B. In this regard, it was the generation of a procedure prediction output based on the claimed robotic surgical procedure weights in connection with a machine learning model that supported reversal of the section 103 rejection.
C. Reversal of Prior Art Rejection, Affirmance of Alice Rejection
Ex parte Trunck, Appeal No. 2022-001298 (PTAB May 11, 2023) involved technology relating to polygenic models that predict characteristics of individuals based on genetic variants of the individuals. Characteristics can include, for example, phenotypes and behaviors of individuals. The claims recited determination of a demographic to which an individual belongs based on genetic variants of the individual. In the claims, a polygenic model from a set of polygenic models in the form of trained machine learning models is selected to make a prediction of a characteristic of the individual. The selected polygenic model, as claimed, has been trained using known genotypes and characteristics for members of the demographic. The claims further recited operation of the selected polygenic model to make the prediction. The applicant appealed rejection of the claims based on sections 101 and 103.
As to the first prong of step 2A, the PTAB found that certain claimed steps could be carried out in the human mind or with pen and paper and that the claimed polygenic prediction server was merely a generic computer. As to the second prong, the PTAB considered the remaining additional elements that recited storing the polygenic models at a polygenic prediction server and operating the selected polygenic model to make the prediction of the characteristic. The PTAB stated that the operating step merely implemented the abstract idea on a computer and concluded that the additional elements did not integrate the abstract idea into a practical application. As to step 2B, the PTAB relied on prior art references cited by the examiner for section 103 rejections to assert that trained machine learning models that predict a characteristic of an individual based on genetic variants of the individual are well-understood, routine, and conventional. The PTAB affirmed the rejection under section 101 but on procedural considerations reversed the rejection under section 103 because the examiner failed to cite specific portions of the prior art references.
Despite its ultimate ruling, the PTAB hinted at a way to potentially bolster subject matter eligibility. In one part of its decision, the PTAB observed that the claims did not recite developing computer models capable of predicting characteristics by applying specific machine learning methods to specific datasets. Rather, according to the PTAB, the claims merely required storing preexisting machine learning models which have already been trained.
III. CONCLUSION
These decisions suggest that PTAB appeals on medical technology incorporating AI are potentially winnable but still unpredictable. In some cases, the scope of issues taken to the PTAB is the same scope considered by it. For example, in Ex parte Lyman, the appeal the applicant wanted on section 103 alone is the appeal the applicant got — and won. Here, and in the other two decisions, the applicant prevailed on its prior art position, perhaps contrasting AI related medical technologies from other crowded fields where reversals may be less common. After the applicant and the examiner reached agreement on Alice, the PTAB did not proactively reconsider the sufficiency of an express recital of training and use of an anatomical location-based machine learning model based on a fine tuning step.
But coming to agreement with an examiner on section 101 issues will not necessarily immunize an appeal from Alice. The APJ in Ex parte Couture did not merely sua sponte raise a new question. Rather, and perhaps to the applicant’s surprise, the APJ went so far as to provide a lengthy explanation as to why the fairly detailed claims on a robotic surgical device and a machine learning engine were purportedly ineligible. Even in a case where two APJs and an examiner agree, one is still left to wonder about the value of a patent issued with invalidity arguments of an APJ prominently set forth in the file history and, more fundamentally, the right circumstances for an appeal that justify such risk.
Of course getting the appeal you want is different from getting the result you want. Based on published statistics, PTAB agreement with the examiner, like the result in Ex parte Trunck, should not be a complete surprise. Given discretion laden realities in application of amorphous Alice principles, the sweet spot with some luck sometimes lies within reach. Although the appeal was lost, the applicant in Ex parte Trunck was fortunate to receive an apparent nudge by the PTAB in a promising direction.
[ad_2]
#Patent #Trial #Appeal #Board #Approaches #Patent #Claims #Medical #Technology #Implementing
Source link
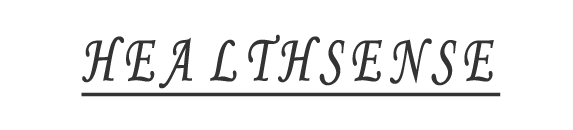